What's wrong with Deterministic forecasting + Top 5 retail forecasting mistakes
50 years ago, life was simpler. You could walk into a store, grab a packet of chips, walk out, and not have to worry about a thing. Now, as soon as you walk into a store, you first have to figure out where the snacks aisle is. If you happen to find it in the first 60 seconds, you have to look over at least 60 different flavours, shapes, and sizes to get to the one you want. By the time you pay and walk out, you’ve been visually influenced to buy 20 other odd, probably useless stuff.
The variety of things available to us has turned our brains into mush, and yet, consumer patterns almost demand this. Back then, retail was simpler, because all retailers had to do was track down sales, plot it all on a graph, and predict future demand. Cut to 2023, when it is unimaginable to do that for each individual item.
The real problem here isn’t the shift in consumer patterns, but the lack of shift in forecasting solutions. While the former has transformed completely, retailers are still being asked to perform exceptionally, while using the same pen and paper method (now called Excel) or other just as simplistic models, like deterministic forecasting solutions. If as a retailer, it feels like you’ve done everything right and still ended up failing, you’re not alone. Multiple retailers face this problem every single day.
We discuss the why, how and what below:
Retail forecasting shouldn’t be a game of guesswork
What’s wrong with deterministic forecasting?
Below, we’ve discussed the top 5 reasons why deterministic forecasting is unsuitable for retail. These are mistakes that retailers often make while making retail decisions that ultimately lead to frustration with the systems and dipping revenue.
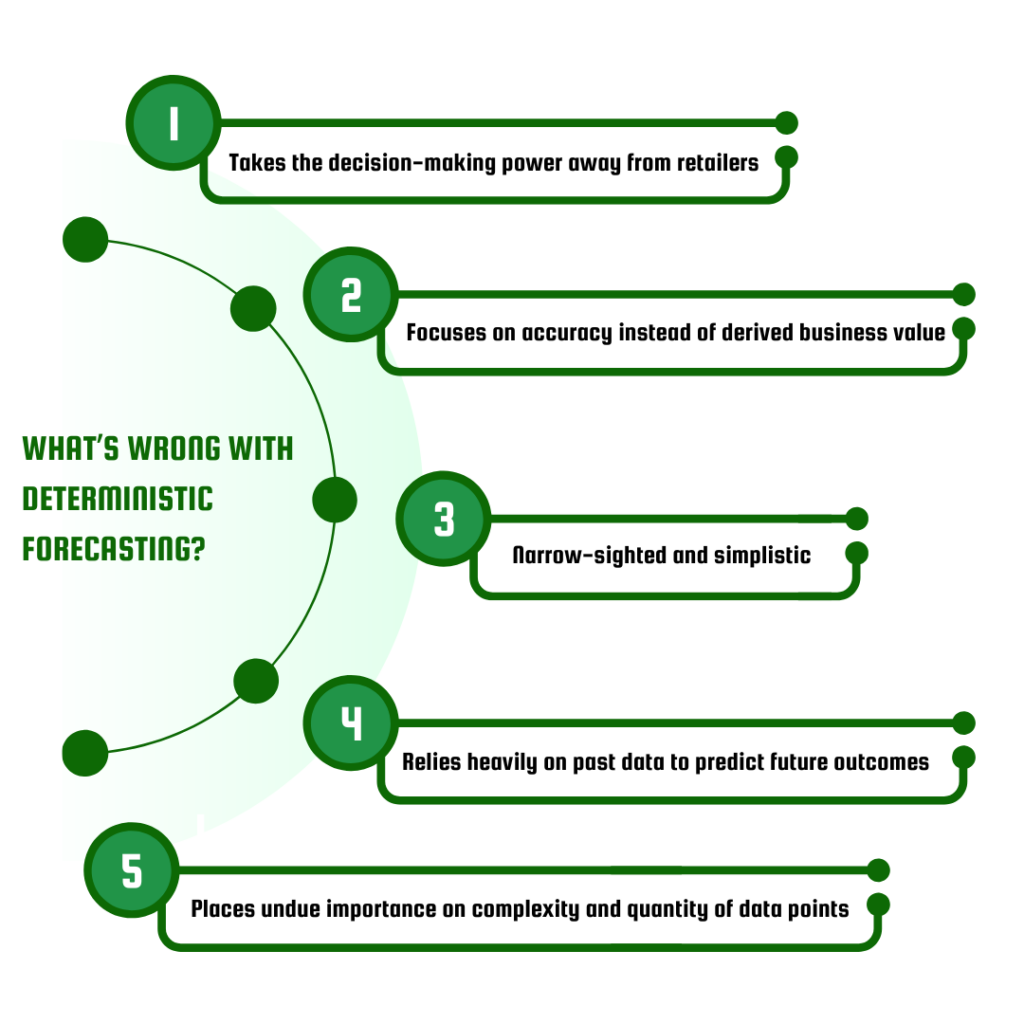
Takes the decision-making power away from retailers
Let’s take a look at a realistic business scenario:
Stacy is the Head of Retail at a 300+ store chain pharmacy. She understands that the real problem they face at work is optimizing the processes in order to decrease stockouts while leaving behind enough inventory to capitalize on upselling and cross-selling opportunities when they present themselves.
- In this case, should she trust a solution that:
Gives her a hyper-accurate result of how to arrange her inventory to minimize costs?
Or, - Gives her the probabilities of stockouts at different inventory levels while accounting for her priority to upsell and cross-sell?
Sure, as a business one of your priorities is cutting costs, but that is not your only priority. You want to expand, grow, diversity, focus on efficiency and so much more. You want to control the steering wheel of your business, so why would you let retail forecasting run on autopilot?
As retailers, it is your responsibility to plan for future demand, why would you give the power away to a solution that accounts for only one possibility from an ocean of probabilities?
Focuses on accuracy instead of derived business value
In business, the things that are easier to measure get measured. One of the key reasons why demand planners obsess over forecasting accuracy is because their performance is graded against a forecast’s performance, which is measured by accuracy.
Assuming in scenario 1, the accuracy of a forecast is 99%. How much impact does this have on the literal business value?
And in scenario 2, the business value is consistently increased by 12% YOY, in this case, does the forecast accuracy even matter?
Unfortunately, businesses assign easily quantifiable KPIs to their demand planners, ultimately hurting not only the team but the organization as a whole.
Forecasting accuracy is merely a means to an end, not the end itself. When we assume accuracy to be the destination, we strive to gain accuracy, without questioning if that is what we truly want. Without changing the mindset that places undue emphasis on accuracy, retailers will continue to struggle.
Narrow-sighted and simplistic
If a football player kicks the football towards the right side of the goal 8 consequent times, can you accurately predict where the ball will be kicked on the 59th time? Of course not, because the answer isn’t that simple. You have to take into account the velocity of the ball, the air pressure, the force used and so many other factors.
You might think that you’d be able to accurately calculate the 9th or the 10th time because of recency bias, but with each extra kick, the uncertainty increases. The biggest problem with using deterministic forecasts in retail is the lack of nuance that it accounts for.
Long-term behaviour is unpredictable even in a perfectly controlled setting as outlined by the billiard ball theory that I talked about on LinkedIn. In a free-will society, this problem snowballs. By simplifying the solution, we are simplifying human behaviour, which is a fundamentally wrong move.
Retail forecasting shouldn’t be a game of guesswork
Relies heavily on past data to predict future outcomes
Another major drawback that is also closely related to the point made above is that deterministic forecasts rely heavily on past data to predict future outcomes. In a controlled environment, this can be true in most parts. But not so much in the real world.
Here’s an example: If a human eats pasta 5 times a week, can you guarantee that they will also eat pasta on the weekend or the next week? If yes, can you tell which type? If it will be overcooked, undercooked, or al dente? If they’ll eat it at 6 pm, 7 pm or at midnight? Simply put, you can’t.
Why? Because humans are unpredictable. They may have eaten pasta for the last 5 days because they had a soon-to-expire pasta box to use, or because they were too tired to whip up something after work, or because their dog was sick and they didn’t have time to meet their own dietary requirements.
Now combine this with the uncertainty of the availability of pasta in the closest supermarket and something beyond our control like the weather. This makes the situation a lot more complicated. In retail, the factors are manifold, so backward-looking forecasts cannot cut it.
Does this imply that the past cannot be reflective of what is to happen in the future? No, but it also cannot be the yardstick you measure the future by, nor can it be an influence on the future. The past simply needs to be looked at as a fact, as something that has happened and is now unchangeable.
Places undue importance on complexity and quantity of data points
A bad workman blames his tools and a bad forecast points at the data. Usually, when forecasts don’t work, retailers assume that it is because they are not feeding the “right” data to the software. While that may be the case sometimes, more often than not, the disappointment in forecasting comes from pursuing the wrong methods.
To solve this problem, retailers resort to collecting more data and collecting data at multiple points. They become “data-obsessed”. While having more data is helpful up until a certain point, collecting data about anything and everything isn’t fruitful in the wrong run.
What good does it do to run the wrong race faster? What is the point of focusing on accuracy when the forecast isn’t designed to benefit you?
In the stock market, even if you plotted multiple points on the past performance of a particular stock, would you ever be able to predict when it falls or rises?
The only 2 options that remain before you are:
- Plot as many points as you humanly can, and be disappointed
- Or, keep an eye out on the market trends, sectorial trends and other variables along with tracking past performance
Surprisingly, the chances of you winning are much higher in the second scenario. Going by Warren Buffet’s quote, “You can never time the markets”.
Similarly, you can’t ever accurately predict what is going to happen, but what you can do is account for all scenarios, and pick the most probable scenarios that best align with your goal(s).
What’s the alternative to deterministic forecasting?
The question that remains unanswered is, “If not deterministic forecasting, then what’s the alternative?”. We’ve already outlined the problems with deterministic forecasting, so the ideal solution meets modern retailers’ requirements. With stochastic forecasting, you:
- Don’t have to rely only on past data to make predictions
- Don’t have to obsess over accuracy
- Can take decisions after considering all probable scenarios
- Can trust a solution that isn’t narrow-sighted
- Have access to a forecast that works all the time, not every so often
We’ve discussed stochastic forecasting at length on our podcast, blog and also my LinkedIn.
Retail forecasting shouldn’t be a game of guesswork
FAQs
1. What is retail forecasting
Forecasting in retail is the process of estimating future customer demand. Ideally, forecasts help retailers make critical business decisions that reduce under & overstocking, improve customer satisfaction rates and help them capitalize on new opportunities.
Retailers can either use a basic, non-specialized software in-house (i.e Excel), or a cloud platform, or outsource forecasting to a specialized service provider to fulfill their forecasting needs.
2. How often does retail sales forecasting software fail?
No forecast is 100% accurate. This is why it is important to focus on the ‘why’ rather than the ‘when’ while dealing with retail forecasts. You can never tell when a forecasting solution will fail, but you can prevent it from being wrong often. It is best to opt for solutions that have proven results, ones that don’t obsess over accuracy but instead focus on derived business value.